Executive summary
Lead prioritization model is a predictive algorithm to score and classify leads. Primarily used by sales organizations to segregate buyers from non-buyers. Model accuracy and implementation effectiveness determine the success of “Lead Prioritization”. Organizations use a threshold-based approach or a descending order approach to target potential customers. Data maturity, risk taking ability and organization’s culture are all key factors in the choice of approach. As market disruptions are constant, effective on-ground implementation, periodic governance and timely model refresh are essential for sustained best results. Apart from focusing on the right leads, marketing can extract target customer profiles from the algorithm to invigorate their lead generation mechanisms. “Lead prioritization” can be extended across functions – Eg: after-sales service – identifying the customers who are likely to need service. Today’s and tomorrow’s business leaders are increasingly harnessing data to power business strategy. Lead prioritization is often the first step to unlocking the potential of predictive analytics. Have you taken the leap?
What is being solved for?
Before becoming a customer, a prospect is part of a sales pipeline in the form of a lead. While leads go through a specific journey to become customers, not all leads end up as one. With the bandwidth of sales teams physically limited, organizations across the world are looking out for intelligent solutions to generate the maximum out of their sales pipelines. A key metric which is tracked across organizations, is lead to sales conversion. Sales and marketing teams which own this metric are constrained by three questions on the path to achieving targeted conversion rates.
- How to ensure every potential customer is attended to? – Minimize opportunity loss
- How to optimize sales force bandwidth and improve effectiveness? – Maximize productivity
- How to generate as many qualified enquiries as possible? – Improved sourcing
“Lead Prioritization” answers these questions while achieving the stated objective of conversion rate improvement.
Why will it be more effective than existing experience-based practices?
Lead prioritization is a methodology to score and target leads basis their business potential (probability of conversion). Sales and marketing teams collect several customer information (demographic, socio-economic, psychographic etc.) at the time of lead generation. In most organizations, teams use one or a few of these data points to target leads. Often, this approach is different across personnel, geographies and largely derived from their past experiences. And almost always, this knowledge and its impact is limited due to one of many reasons
- Knowledge is restricted to the user’s intuition
- Knowledge is restricted for reasons of internal competitiveness
- Decision making does not include all necessary variables and hence the impact is muted
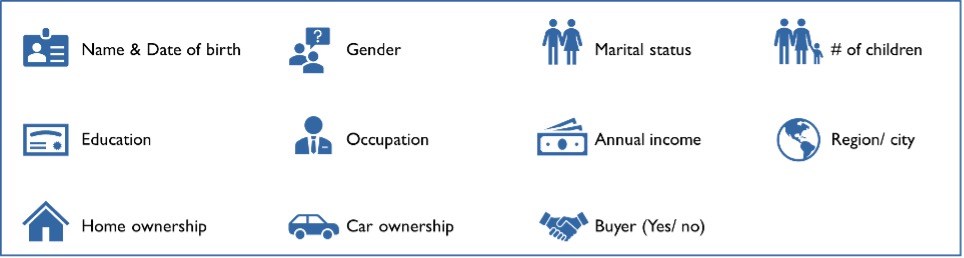
Fig 1: Sample customer information collected during lead generation
Implementation of a “Lead prioritization” algorithm overcomes all these challenges and institutionalizes a data driven decision making culture.
What is a lead prioritization algorithm?
At its heart, the predictive algorithm analyses past data (old sales pipeline) to draw customer profiles, compares them with the current sales pipeline and “scores” each lead. Lead “score” reflects the probability of a lead to convert to a sale.
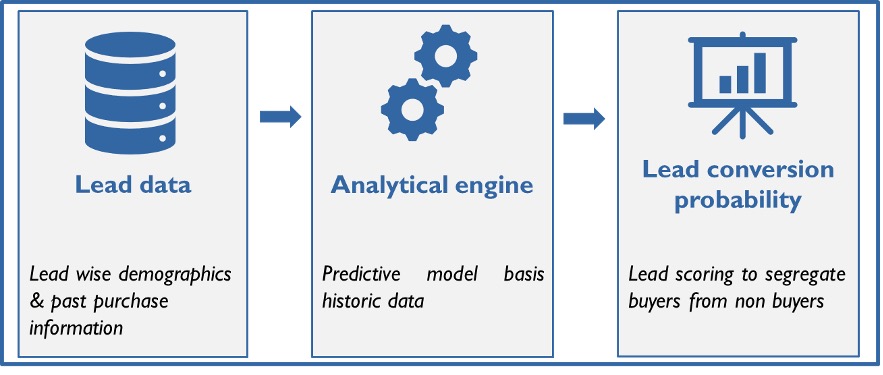
Fig 2: Lead prioritization algorithm working
In our experience implementing this algorithm, the best results have been obtained when the model receives real-time feedback and the prediction equation is fine tuned constantly on a periodic basis. This is primarily because customer needs, aspirations and market forces all change over time. A model based on one-time analysis cannot keep pace with these changes. Effective organizations engage constantly with the algorithm. They have a robust review system with scorecards and feedback loops to evaluate the model performance, assimilate new variables and drop ineffective variable to calibrate the algorithm to perfection.
The success or failure of the lead prioritization algorithm is a combination of 2 elements – the accuracy of prediction and the effectiveness of its use in decision making. It is common to see accuracies in the range of 70% at the time of initiation. Model accuracy is sharpened over time with real-time feedback and improved to ~90% and above.
In our experience implementing this algorithm, the best results have been obtained when the model receives real-time feedback and the prediction equation is finetuned constantly on a periodic basis. This is primarily because customer needs and aspirations and market forces all change over time and a model based on one-time analysis cannot keep pace with these changes.
Effective organizations engage constantly with the algorithm. They have a robust review system with scorecards and feedback loops to evaluate the model performance, assimilate new variables and drop ineffective variable to calibrate the algorithm to perfection.
Ok, so now that leads have been scored, what next?
Two approaches are widely practiced. Organization’s culture is critical in determining the most suitable approach
Approach 1: Threshold based
This approach uses the lead scores to bucket them into 2 distinct categories – Buyers and Non-buyers. A score threshold is administered to aggregate leads into “Buyer” or “Non-buyer” categories. The success or failure of this model is determined by its accuracy – What is the % of true positives (actual buyers who are classified as buyers) and true negatives (actual non-buyers who are classified as non-buyers)?

The approach instructs the sales teams to specifically focus on one group to get maximum results – while deprioritizing or in some cases neglecting the other group. The model’s capture rate, which determines what % of total buyers have been captured is another important metric to measure the effectiveness of the algorithm.

Organizations should be wary of neglecting one group to uniquely focus on the other. This approach can create a “self-fulfilling prophecy”, thereby losing out on critical feedback from the group classified as “non-buyers” to sharpen the algorithm over time.
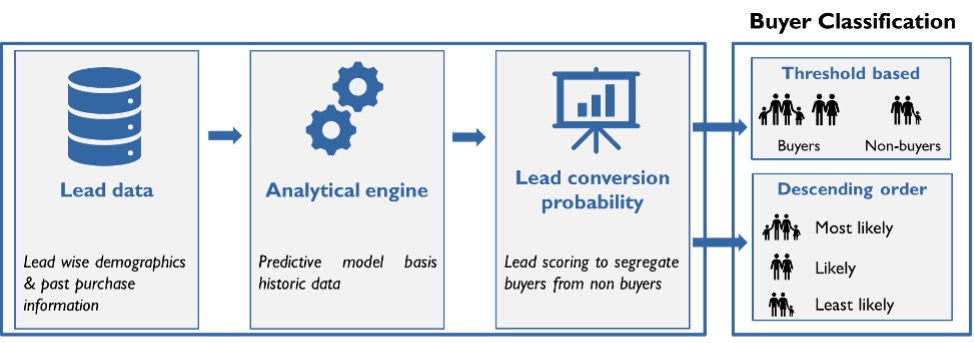
Fig 3: Buyer classification approaches
Approach 2: Descending order
A less risky alternative is to rank and order the leads in the descending order of their scores. It advises the sales teams to order their time and effort basis the predicted scores. This approach is favored by more risk averse organizations as it ensures there is no short-term loss in sale as all leads will be attended to. On the contrary this approach may not make the most judicious use of available sales and marketing resources.
This approach measures conversion and capture rates to evaluate algorithm performance
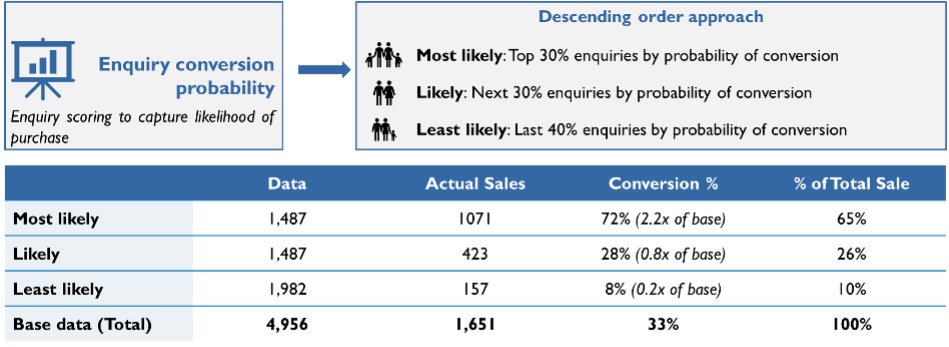
Fig 4: Illustrative chart on implementation of “Descending order” approach
Organizations, who are lower on data maturity, also tend to take this approach. This awards them the necessary time to sharpen the quality of their data. And in the long-term business leaders have been seen to favor the “threshold based” method.
What can marketing teams take from this model?
While leads are scored and categorized as “buyers” and “non-buyers”, this classification also helps marketing teams determine the “ideal target customer profile”. The target customer is a combination of the same demographic, socio-economic and psychographic data points used to predict buying behavior. While, the algorithm at the backend, uses this profile to match it with new data and predict their probabilities of conversion, effective organizations use this further to their advantage. Marketing heads extract this intelligence and align their team’s efforts towards campaigns and activations that generate leads most similar to the “ideal target customer profile”. This way, the marketing spend RoI is improved while organically improving sales conversions.
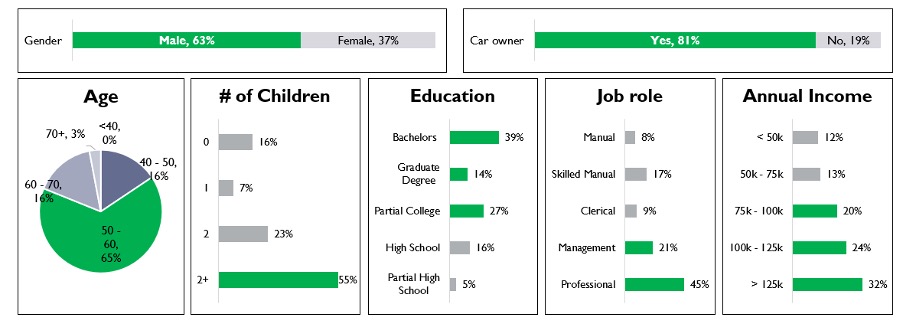
Fig 5: Illustrative target customer profile
Is this algorithm only applicable to sales and marketing?
While, the example of sales has been used to illustrate the lead prioritization algorithm, the use cases are plenty. Functions within an organization can extend the recommendation algorithm to improve efficiency in their roles. Eg: Procurement managers can estimate vendors risk probabilities before deciding on whom to engage with.
Cross industry application is equally possible. Financial organizations have been able to pre-empt frauds and avoid bad debts by rejigging the predictor variable accordingly. After-sales service professionals have delighted customers by forecasting their arrival at workshops and customizing the entire journey – from welcome to vehicle delivery – to their tastes.
Inter-function and inter-industry application of the lead prioritization algorithm is on the rise in the west. And India is not being left behind. Data challenges remain – as the model’s effectiveness is predicated significantly on quality of data. Organizations have begun to understand the benefits that are possible and what they are missing out on by not focusing on data. Higher investments are being seen in data architecture to capture relevant data. Future ready organizations are increasingly investing in predictive algorithms to drive business decisions – and lead prioritization is often the starting point. So, let us leave you with 2 questions. Does your business proactively collect and use data to take business decisions? By now you would have drawn parallels to your organization. So, where and how do you think lead prioritization can fit in?
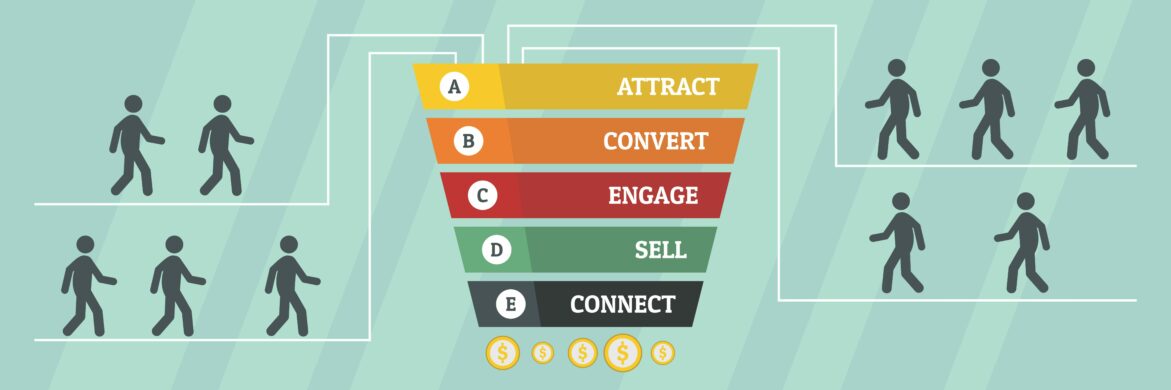